阅读:0
听报道
撰文 | Frank Wilczek
翻译 | 胡风、梁丁当
中文版
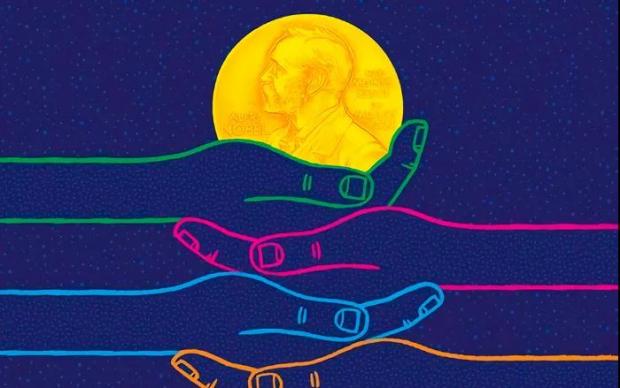
从明确科学发现的贡献度到训练人工智能思考,在科学界,如何奖励成功是一项重要却十分困难的任务。
20世纪70年代末、80年代初是一个令人兴奋的时代。当时,包括我在内的众多物理学家都信心满满地认为,我们即将实现基本力的大统一理论。这个理论的一个惊人预言是:质子是不稳定的,它像许多放射性核一样,最终也会衰变。人们普遍期待着实验物理学家能够找到验证这个预言的办法。
他们确实做到了。可不幸的是,这些声称观察到质子衰变的实验被后来的工作证明是错的,尽管错误的性质一直没有完全澄清。
这样的故事屡见不鲜。近年来,不断有研究宣称发现了某个奇特的物理现象——包括磁单极、宇宙暗物质、轴子和超对称粒子等等,可随后,这些结论都被灵敏度更高的实验否定了。
假如将来的研究真的发现了质子衰变或其他效应,从而证明早期实验仓促得出的结论是对的,那这些工作就会荣获大奖。
想到可能出现的混乱场面,我有个异想天开的提议:设立一个反诺贝尔奖。
这个奖项会授予这类研究工作,即如果这项工作是正确的,那么它值得一个诺贝尔奖。为了不让人难堪,颁奖会秘密进行。这个奖只有在获得者后来做出了其他值得获得诺奖的工作时才会发挥作用——两个奖将彼此抵消。这样的前景或许会让那些过于野心勃勃、轻率的科学家们变得稍微谨慎一些。
在取得成功后,贡献大小的确认和奖励的分配是科学社会学中的一大难题。一项工作的成功显然离不开很多人在方方面面的贡献,但是奖项、优厚的职位和丰厚的知识产权却只能授予少数个体。用物理学的术语来说,就是奖励是“量子化”的,要么有要么无。但是贡献的方式与大小却是多元化的。
金钱是一项伟大的发明。相比于以物易物的方式,它能够更灵活和更具辨识度地实现利益的分配。但是,众所周知,金钱本身并不能解决所有关于公平和有效分配的问题。
在学习中,如何分配贡献度也是一个关键问题。神经网络,无论是生物的还是人工的,都希望“奖励”(即加强)那些有助于成功的联系,同时“惩罚”那些收效甚微或导致失败的联系。这种联系的强度可以连续变化,从而避免了奖励全有或全无的量子化现象。
但在通常情况下,任何决策活动都涉及到很多的神经关联。因此,我们仍然需要解决如何论功行赏,或是在出错时如何分担责任的问题。
由此涌现出了一些非常聪明的工作,例如,深度学习神经网络在模式识别领域取得了让人惊叹的成功,它还在象棋、围棋、魔兽世界这些复杂的游戏中表现优异,甚至超越最顶级的人类玩家。
总有一天,人工智能(AI)会成为——如果我们能教会它们智慧的话——能划分贡献度的有力工具。将来,当AI的研究获得诺贝尔奖时,势必会有一堆这样的棘手问题,而AI恰好可以帮助我们。
英文版
From assigning credit for discoveries to teaching AIs to think, rewarding success is a crucial but difficult task for science.
In the heady days of the late 1970s and early 1980s, many physicists-including me-thought they were on the cusp of achieving a unified theory of the fundamental forces. A striking prediction to emerge from this circle of ideas is that protons are unstable and will eventually decay, just as many radioactive nuclei do. It was widely hoped that experimenters would find ways to verify the prediction.
Sure enough, they did. Unfortunately, subsequent work revealed that the claimed observations of proton decay could not be correct, though the nature of the experiments’ flaws was never clarified completely.
This story is not unique: In recent years a number of exotic physical phenomena—including magnetic monopoles, cosmological dark matter, axions and supersymmetric particles—have reportedly been detected, only for later, more sensitive experiments to come up empty.
If later work had actually discovered proton decay, or the other effects, those who jumped the gun might have seemed vindicated and then come up for big rewards.
Musing on these potential messes, I came up with a whimsical suggestion: the anti-Nobel prize.
An anti-Nobel would be awarded for incorrect work that, had it been correct, would have merited a Nobel Prize. It would be awarded secretly, so no one need be embarrassed. The anti-Nobel prize would only come into play if the recipient did subsequent Prize-worthy work, in which case, the two would cancel each other out. This prospect might give overly ambitious, trigger-happy scientists some pause.
The problem of assigning credit and rewards for success is a big issue in the sociology of science. Prizes, plum positions and lucrative intellectual property rights can only be awarded to a few individuals, even when the underlying work involves, at different levels, many contributors. To use a physics term, the rewards are “quantized,” given on an all-or-nothing basis, while the contributions come in varied shapes and sizes.
Money is a great invention that allows rewards for economic effort to be divided up with more flexibility and discernment than barter. Notoriously, though, that breakthrough by itself doesn’t solve all problems of fair and efficient distribution.
The credit assignment problem is also a central issue in learning. Within neural networks, natural or artificial, one wants to “reward”—that is, strengthen—connections that are involved in successful outcomes, while “punishing” those that accomplish little or lead to failures. Since the strength of connections can vary continuously, one can avoid the all-or-nothing quantization of credit.
Typically, however, any decision or activity involves many neural connections, so the problem of apportioning credit for success and blame for failure must still be addressed.
Some very clever work is being done on this: Deep learning neural networks have had impressive successes in learning to identify patterns and play difficult games including chess, Go and World of Warcraft extremely well.
One day, AIs will be powerful tools in assigning credit wisely (if, that is, we can teach them wisdom). We’ll need their help in dealing with the knotty issues sure to arise around awarding Nobel prizes to AIs.
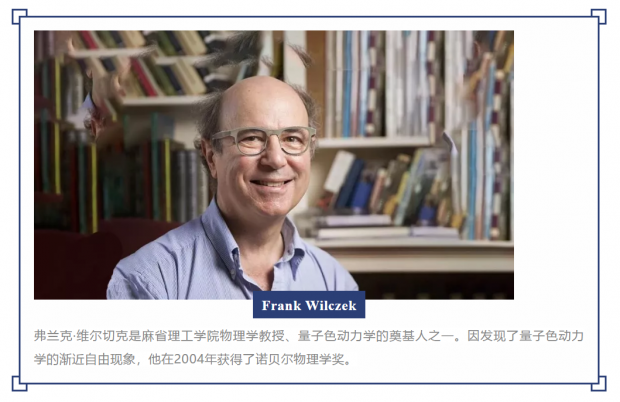
本文经授权转载自微信公众号“蔻享学术”。
话题:
0
推荐
财新博客版权声明:财新博客所发布文章及图片之版权属博主本人及/或相关权利人所有,未经博主及/或相关权利人单独授权,任何网站、平面媒体不得予以转载。财新网对相关媒体的网站信息内容转载授权并不包括财新博客的文章及图片。博客文章均为作者个人观点,不代表财新网的立场和观点。